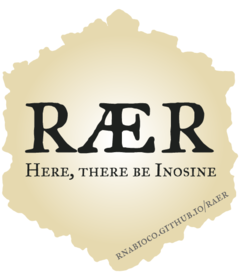
Identifying RNA editing sites using RNA and DNA sequencing data
Kent Riemondy
University of Colorado School of Medicine2023-09-20
Source:vignettes/novel-sites.Rmd
novel-sites.Rmd
library(raer)
library(GenomicFeatures)
library(SummarizedExperiment)
library(rtracklayer)
library(TxDb.Hsapiens.UCSC.hg38.knownGene)
Novel RNA editing site detection tutorial
This vignette will demonstrate how to identify novel RNA editing
sites using the raer
package.
In this vignette a public RNA-seq and Whole-Genome sequencing dataset will be analyzed. High coverage whole-genome sequencing was conducted ERR262997 along with paired-end RNA-seq SRR1258218.
Aligned BAM files and a genome fasta file have been preprocessed for
this vignette. These files occupy ~100 Mb of space and contain
alignments from the first 1MB of chromosome 4 and they are stored in a
BiocFileCache
.
fns <- download_NA12878()
fns
## $bams
## [1] "/github/home/.cache/R/raer/ERR262996_dedup_chr4_sub.bam"
## [2] "/github/home/.cache/R/raer/SRR1258218_Aligned.sorted.dedup_sub.bam"
##
## $bai
## [1] "/github/home/.cache/R/raer/ERR262996_dedup_chr4_sub.bam.bai"
## [2] "/github/home/.cache/R/raer/SRR1258218_Aligned.sorted.dedup_sub.bam.bai"
##
## $fasta
## [1] "/github/home/.cache/R/raer/5c715cfbfb8_hg38_chr4.fa.bgz"
##
## $snps
## [1] "/github/home/.cache/R/raer/5c772bffd8c_chr4snps.bed.gz"
##
## $rmsk
## [1] "/github/home/.cache/R/raer/5c71936face_rmsk_hg38.tsv.gz"
Additionally we will use the following additional annotation resources:
- A database of known SNPs, for example the
SNPlocs.Hsapiens.dbSNP155.GRCh38
package. Due to space and memory constraints in this vignette we will use a BED file containing SNPs from the first 1Mb region of chr4.
-
TxDb.Hsapiens.UCSC.hg38.knownGene
, a database of transcript models. Alternatively these can be generated from a.gtf
file usingmakeTxDbFromGRanges()
from theGenomicFeatures
package.
- RepeatMasker annotations, which can be obtained from the
AnnotationHub()
for hg38. For space reasons these annotations for chr4 have been included as a text file.
txdb <- TxDb.Hsapiens.UCSC.hg38.knownGene
chr4snps <- import(fns$snps)
rmskhg38 <- read.table(fns$rmsk)
rmskhg38 <- makeGRangesFromDataFrame(rmskhg38,
keep.extra.columns = TRUE,
starts.in.df.are.0based = TRUE
)
The pileup_sites()
function can accept multiple bam
files, here we supply one from RNA-seq, and one from whole genome
sequencing.
wgs_bam <- fns$bams[1]
rna_bam <- fns$bams[2]
fafn <- fns$fasta
Filtering parameters for the pileup_sites()
function can
accept multiple arguments matched to the input bam files.
fp <- FilterParam(
min_depth = 1,
min_base_quality = 30,
min_mapq = c(255, 30),
library_type = c("fr-first-strand", "unstranded"),
trim_5p = 5,
trim_3p = 5,
indel_dist = 4,
homopolymer_len = 6,
max_mismatch_type = c(3, 3),
read_bqual = c(0.25, 20),
only_keep_variants = c(TRUE, FALSE)
)
bams <- c(rna_bam, wgs_bam)
names(bams) <- c("rna", "dna")
rse <- pileup_sites(bams,
fasta = fafn,
chrom = "chr4",
param = fp
)
rse
## class: RangedSummarizedExperiment
## dim: 546 2
## metadata(0):
## assays(7): ALT nRef ... nC nG
## rownames(546): site_chr4_44338_2 site_chr4_124820_1 ...
## site_chr4_990981_1 site_chr4_993342_1
## rowData names(4): REF rpbz vdb sor
## colnames(2): rna dna
## colData names(1): sample
Next we filter to keep those sites with a variant in the RNA-seq, but
no variant in the DNA-seq, and a minimum of 5 reads in the DNA-seq. The
DNA-seq data will be reported on the “+” strand, where as the RNA-seq
data will be reported on either strand. We therefore use
subsetByOverlaps(..., ignore.strand = TRUE)
to retain sites
passing these DNA-seq based filters independent of strand.
to_keep <- (assay(rse, "nRef")[, "dna"] >= 5 & assay(rse, "ALT")[, "dna"] == "-")
rse <- subsetByOverlaps(rse, rse[to_keep, ], ignore.strand = TRUE)
nrow(rse)
## [1] 198
Next we filter to remove any multi-allelic sites. These sites are
stored as comma-separated strings in the ALT
assay
(e.g. G,C
). Non-variant sites are stored as -
.
filter_multiallelic()
will remove any sites that have
multiple variants in the samples present in the
summarizedExperiment
object. It will add a new column to
the rowData()
to indicate the variant for each site, and
will calculate an allele_freq
assay with variant allele
frequencies for each sample.
rse <- filter_multiallelic(rse)
## ℹ `filter_multiallelic()`: removed 0 sites from 198 (198 remain)
rse <- calc_edit_frequency(rse)
## ℹ 48 sites had no coverage for calculating editing
rowData(rse)
## DataFrame with 150 rows and 5 columns
## REF rpbz vdb sor ALT
## <character> <numeric> <numeric> <numeric> <character>
## site_chr4_124820_1 C -0.939623 Inf 1.410919 A
## site_chr4_125213_1 G -1.584745 Inf 0.367725 C
## site_chr4_126885_1 A 0.826528 Inf 2.271553 G
## site_chr4_126955_1 A 1.584745 Inf 1.506342 G
## site_chr4_134331_1 A -1.868257 0.1 0.367725 G
## ... ... ... ... ... ...
## site_chr4_894077_1 G 1.289956 Inf 1.524254 A
## site_chr4_952419_1 G -1.362512 Inf 0.550831 C
## site_chr4_958447_1 C 0.694986 Inf 1.436657 A
## site_chr4_990589_1 A -1.640049 Inf 1.570697 C
## site_chr4_993342_1 A 1.498680 Inf 1.472727 G
Next we’ll remove sites in simple repeat regions. We will add repeat
information to the rowData()
using the
annot_from_gr()
function.
rse <- annot_from_gr(rse, rmskhg38, cols_to_map = c(c("repName", "repClass", "repFamily")))
rowData(rse)[c("repName", "repFamily")]
## DataFrame with 150 rows and 2 columns
## repName repFamily
## <Rle> <Rle>
## site_chr4_124820_1 NA NA
## site_chr4_125213_1 MER21C ERVL
## site_chr4_126885_1 NA NA
## site_chr4_126955_1 NA NA
## site_chr4_134331_1 NA NA
## ... ... ...
## site_chr4_894077_1 NA NA
## site_chr4_952419_1 NA NA
## site_chr4_958447_1 NA NA
## site_chr4_990589_1 NA NA
## site_chr4_993342_1 NA NA
Next we’ll remove sites adjacent to other sites with different variant types. For example if an A->G variant is located proximal to a C->T variant then the variants will be removed.
rse <- filter_clustered_variants(rse, txdb, variant_dist = 100)
## ℹ `filter_clustered_variants()`: removed 22 sites from 149 (127 remain)
rse
## class: RangedSummarizedExperiment
## dim: 127 2
## metadata(0):
## assays(9): ALT nRef ... depth edit_freq
## rownames(127): site_chr4_124820_1 site_chr4_125213_1 ...
## site_chr4_990589_1 site_chr4_993342_1
## rowData names(8): REF rpbz ... repClass repFamily
## colnames(2): rna dna
## colData names(3): sample n_sites edit_idx
Next, sites within 4bp of known splicing events will be excluded. These sites are low-confidence due to the possibility of mis-alignments near splicing events.
rse <- filter_splice_variants(rse, txdb)
## ℹ `filter_splice_variants()`: removed 2 sites from 127 (125 remain)
rse
## class: RangedSummarizedExperiment
## dim: 125 2
## metadata(0):
## assays(9): ALT nRef ... depth edit_freq
## rownames(125): site_chr4_124820_1 site_chr4_125213_1 ...
## site_chr4_990589_1 site_chr4_993342_1
## rowData names(8): REF rpbz ... repClass repFamily
## colnames(2): rna dna
## colData names(3): sample n_sites edit_idx
Next, we’ll annotate if the site is a known SNP and remove any known
SNPs. If using a SNPlocs package you can use the
annot_snp()
function. However we will use the
annot_from_gr()
function to annotate using the prebuilt
chr4snps
object.
rse <- annot_from_gr(rse, chr4snps, "name")
rowData(rse)[c("name")]
## DataFrame with 125 rows and 1 column
## name
## <Rle>
## site_chr4_124820_1 rs1553807640
## site_chr4_125213_1 rs1286128437
## site_chr4_126885_1 NA
## site_chr4_126955_1 NA
## site_chr4_134331_1 rs1715464292
## ... ...
## site_chr4_894077_1 NA
## site_chr4_952419_1 rs1577433212
## site_chr4_958447_1 rs1052651854
## site_chr4_990589_1 NA
## site_chr4_993342_1 NA
## class: RangedSummarizedExperiment
## dim: 78 2
## metadata(0):
## assays(9): ALT nRef ... depth edit_freq
## rownames(78): site_chr4_126885_1 site_chr4_126955_1 ...
## site_chr4_990589_1 site_chr4_993342_1
## rowData names(9): REF rpbz ... repFamily name
## colnames(2): rna dna
## colData names(3): sample n_sites edit_idx
Lastly, we’ll further filter the edit sites to require that the editing frequency is > 0.05 and that at least 2 reads support the editing site.
to_keep <- assay(rse, "edit_freq")[, 1] > 0.05
rse <- rse[to_keep, ]
rse <- rse[assay(rse, "nAlt")[, 1] >= 2]
## GRanges object with 12 ranges and 9 metadata columns:
## seqnames ranges strand | REF rpbz
## <Rle> <IRanges> <Rle> | <character> <numeric>
## site_chr4_134353_1 chr4 134353 + | A -1.1811989
## site_chr4_377951_1 chr4 377951 + | A 0.3595421
## site_chr4_378004_1 chr4 378004 + | A -1.9668785
## site_chr4_378011_1 chr4 378011 + | A -0.0481455
## site_chr4_378018_1 chr4 378018 + | A -0.3099735
## ... ... ... ... . ... ...
## site_chr4_378368_1 chr4 378368 + | A 1.096651
## site_chr4_378409_1 chr4 378409 + | A 0.731101
## site_chr4_380392_1 chr4 380392 + | A 0.332543
## site_chr4_778739_2 chr4 778739 - | A 0.577350
## site_chr4_778780_2 chr4 778780 - | A -0.866025
## vdb sor ALT repName repClass
## <numeric> <numeric> <character> <Rle> <Rle>
## site_chr4_134353_1 0.100000 0.527355 G <NA> <NA>
## site_chr4_377951_1 0.580000 2.285077 G AluSc SINE
## site_chr4_378004_1 0.060000 0.464813 G AluSc SINE
## site_chr4_378011_1 0.840000 0.550831 G AluSc SINE
## site_chr4_378018_1 0.535497 1.070610 G AluSc SINE
## ... ... ... ... ... ...
## site_chr4_378368_1 0.180000 0.268990 G <NA> <NA>
## site_chr4_378409_1 0.580000 0.891998 G <NA> <NA>
## site_chr4_380392_1 0.520000 0.424565 G AluSq2 SINE
## site_chr4_778739_2 0.230191 0.668541 G AluSg SINE
## site_chr4_778780_2 0.303109 1.885595 G AluSg SINE
## repFamily name
## <Rle> <Rle>
## site_chr4_134353_1 <NA> <NA>
## site_chr4_377951_1 Alu <NA>
## site_chr4_378004_1 Alu <NA>
## site_chr4_378011_1 Alu <NA>
## site_chr4_378018_1 Alu <NA>
## ... ... ...
## site_chr4_378368_1 <NA> <NA>
## site_chr4_378409_1 <NA> <NA>
## site_chr4_380392_1 Alu <NA>
## site_chr4_778739_2 Alu <NA>
## site_chr4_778780_2 Alu <NA>
## -------
## seqinfo: 194 sequences from an unspecified genome
Session info
## R version 4.3.1 (2023-06-16)
## Platform: x86_64-pc-linux-gnu (64-bit)
## Running under: Ubuntu 22.04.3 LTS
##
## Matrix products: default
## BLAS: /usr/lib/x86_64-linux-gnu/openblas-pthread/libblas.so.3
## LAPACK: /usr/lib/x86_64-linux-gnu/openblas-pthread/libopenblasp-r0.3.20.so; LAPACK version 3.10.0
##
## locale:
## [1] LC_CTYPE=en_US.UTF-8 LC_NUMERIC=C
## [3] LC_TIME=en_US.UTF-8 LC_COLLATE=en_US.UTF-8
## [5] LC_MONETARY=en_US.UTF-8 LC_MESSAGES=en_US.UTF-8
## [7] LC_PAPER=en_US.UTF-8 LC_NAME=C
## [9] LC_ADDRESS=C LC_TELEPHONE=C
## [11] LC_MEASUREMENT=en_US.UTF-8 LC_IDENTIFICATION=C
##
## time zone: UTC
## tzcode source: system (glibc)
##
## attached base packages:
## [1] stats4 stats graphics grDevices utils datasets methods
## [8] base
##
## other attached packages:
## [1] TxDb.Hsapiens.UCSC.hg38.knownGene_3.17.0
## [2] rtracklayer_1.60.1
## [3] SummarizedExperiment_1.30.2
## [4] MatrixGenerics_1.12.3
## [5] matrixStats_1.0.0
## [6] GenomicFeatures_1.52.2
## [7] AnnotationDbi_1.62.2
## [8] Biobase_2.60.0
## [9] GenomicRanges_1.52.0
## [10] GenomeInfoDb_1.36.3
## [11] IRanges_2.34.1
## [12] S4Vectors_0.38.1
## [13] BiocGenerics_0.46.0
## [14] raer_0.99.11
## [15] BiocStyle_2.28.1
##
## loaded via a namespace (and not attached):
## [1] tidyselect_1.2.0 dplyr_1.1.3
## [3] blob_1.2.4 R.utils_2.12.2
## [5] filelock_1.0.2 Biostrings_2.68.1
## [7] bitops_1.0-7 SingleCellExperiment_1.22.0
## [9] fastmap_1.1.1 RCurl_1.98-1.12
## [11] BiocFileCache_2.8.0 GenomicAlignments_1.36.0
## [13] XML_3.99-0.14 digest_0.6.33
## [15] lifecycle_1.0.3 KEGGREST_1.40.0
## [17] RSQLite_2.3.1 magrittr_2.0.3
## [19] compiler_4.3.1 progress_1.2.2
## [21] rlang_1.1.1 sass_0.4.7
## [23] tools_4.3.1 utf8_1.2.3
## [25] yaml_2.3.7 data.table_1.14.8
## [27] knitr_1.44 prettyunits_1.1.1
## [29] S4Arrays_1.0.6 bit_4.0.5
## [31] curl_5.0.2 DelayedArray_0.26.7
## [33] xml2_1.3.5 abind_1.4-5
## [35] BiocParallel_1.34.2 withr_2.5.0
## [37] purrr_1.0.2 R.oo_1.25.0
## [39] desc_1.4.2 grid_4.3.1
## [41] fansi_1.0.4 biomaRt_2.56.1
## [43] cli_3.6.1 rmarkdown_2.25
## [45] crayon_1.5.2 ragg_1.2.5
## [47] generics_0.1.3 httr_1.4.7
## [49] rjson_0.2.21 DBI_1.1.3
## [51] cachem_1.0.8 stringr_1.5.0
## [53] zlibbioc_1.46.0 parallel_4.3.1
## [55] BiocManager_1.30.22 XVector_0.40.0
## [57] restfulr_0.0.15 vctrs_0.6.3
## [59] Matrix_1.6-1.1 jsonlite_1.8.7
## [61] bookdown_0.35 hms_1.1.3
## [63] bit64_4.0.5 systemfonts_1.0.4
## [65] jquerylib_0.1.4 glue_1.6.2
## [67] pkgdown_2.0.7 codetools_0.2-19
## [69] stringi_1.7.12 BiocIO_1.10.0
## [71] tibble_3.2.1 pillar_1.9.0
## [73] rappdirs_0.3.3 htmltools_0.5.6
## [75] GenomeInfoDbData_1.2.10 BSgenome_1.68.0
## [77] R6_2.5.1 dbplyr_2.3.3
## [79] textshaping_0.3.6 rprojroot_2.0.3
## [81] evaluate_0.21 lattice_0.21-8
## [83] R.methodsS3_1.8.2 png_0.1-8
## [85] Rsamtools_2.16.0 memoise_2.0.1
## [87] bslib_0.5.1 xfun_0.40
## [89] fs_1.6.3 pkgconfig_2.0.3